Projects:LesionSegmentation
Back to Utah 2 Algorithms
Lesion Segmentation
Description
Quantification, analysis and display of brain pathology such as white matter lesions as observed in MRI is important for diagnosis, monitoring of disease progression, improved understanding of pathological processes and for developing new therapies. Utah Center for Neuroimage Analysis develops new methodology for extraction of brain lesions from volumetric MRI scans and for characterization of lesion patterns over time. The images show white matter lesions (yellow) displayed with ventricles (blue) and transparent brain surface in a patient with an autoimmune disease (lupus). Lesions in white matter and possible correlations with cognitive deficits are also studied in patients with multiple sclerosis (MS), chronic depression, Alzheimer’s disease (AD) and in older persons.
In addition to the identification of the location and shape of lesions in 3D, we are interested in analyzing the longitudinal series of brain MRI showing lesions. For this purpose, we have developed a method for estimating a physical model for lesion formation. The model that we use is an approximation using a reaction-diffusion process that is based on expected diffusion properties (as observed through DTI). This approach gives a richer parametrization of lesion changes in addition to volume and location, as the model estimation provides descriptions of growth and spread for individual lesions. In the future, we plan to incorporate this approach for analyzing lesion MRI of a subject over time by characterizing the change patterns through the physical model parameters.
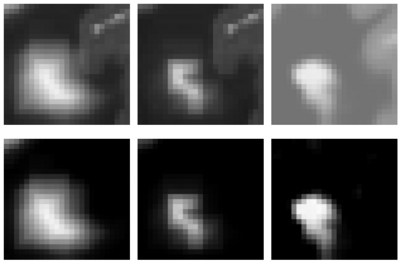
Key Investigators
- Utah Algorithms: Marcel Prastawa, Guido Gerig
- Clinical Collaborators
- MIND: Jeremy Bockholt, Mark Scully
Publications
In Print